Blog
Top 4 Questions to Ask AI Vendors

The pace of AI developments doesn't seem to be slowing anytime soon. With every new breakthrough, the enterprise AI market gets savvier and savvier. One symptom of this growing market is the trend of "AI washing," where the term "AI" continues to be used by marketers to package products that look like AI but are actually "if this, then that" rule-based systems.
To make matters more confusing for the buyer, the first string of decision-makers usually aren't AI experts. It can be difficult for them to understand what's happening under the hood, especially in crowded markets with seemingly similar solutions.
If you're new to AI, be sure to raise these four questions the next time you have to vet an AI vendor.
1. How much user effort is required during onboarding? What about regular ongoing user interactions?
The best AI products come pre-trained and are built for out-of-the-box performance and scalability.
Ask the vendor if the product is ready to go on day one without any programming from the user to provide accurate results. Look for answers that describe a quick onboarding process. If the vendor is truly offering pre-trained AI, it should only be a matter of days or weeks to get the platform up and running.
A reliable AI product requires minimal ongoing user interaction. The only way the user needs to be involved is if they periodically want to help the AI get smarter. While the AI should work well out of the box, it's still helpful to have a feedback loop where the user can lightly tune their own models. Thoughtfully designed AI products will offer a built-in feedback loop that allows users to validate certain outputs or invalidate false positives or false negatives.
There should be less tuning involved as the AI becomes more accurate, and the product should always limit the potential for user impact. Users can inadvertently degrade the model by training it in the wrong direction — causing the AI to return increasingly inaccurate predictions. This is called "model drift" and often happens when a model is overfit on new data and stops working on data it used to work well on.
You won't worry much about model drift with a well-designed AI product. Best-of-breed products will be able to balance the old and new data in a way that doesn't break historical data while still improving for new variables.
2. Will the AI get smarter and more accurate over time?
While you're on the topic of feedback loops, that's a good time to find out whether the product uses advanced machine learning or a rule-based or RPA system.
Rule-based systems basically use "if-then" logic. You can refine the rules, making them more complex or more sophisticated, but it will always be a deterministic scenario. You can't give feedback to rule-based systems, which means the model doesn't get much smarter.
By contrast, deep learning AI products are expected to learn and improve over time because their models are consistently trained and re-trained.
An AI vendor should perform real-time monitoring of accuracy metrics to make sure that the models stay above certain benchmarks, which will vary by industry. For example, in the contract management space where I work, the precision and recall of AI models to extract key information from contracts should always be above 90% or 95%.
As the user engages with the solution, such as updating data or overriding the AI, the vendor should keep monitoring that accuracy. If it drops, the software should trigger a re-training of the user's models on the new data to raise it back to the original success metric.
3. Will the AI work on data it hasn't been trained on before?
Look for answers that explain how their system works with use cases or content it's never seen before, or how it works across different industries and verticals. For example, in contract management, the best solutions work well across all contract types. That includes third-party paper or new types of contracts.
Do a live demo and bring your own content to judge the data extraction in real-time. Be wary if they ask for the content too many days in advance, which is a sign of a possible data-entry farm and not a true AI solution.
4. How much visibility will I have into the AI?
Good vendors make it easy for you to validate the quality of the data or the AI outputs. Platforms that show you where the extracted data comes from make it much quicker for your team to review and pass a judgement on whether the output is correct. Forgoing that QC process defeats the purpose of having the AI in the first place — you're still having to manually review all the materials to verify the AI's accuracy.
Conclusion
If you take nothing else away from this article, remember the value of a live demo. You get the chance to put the vendor in the hot seat to prove they can meet your specific requirements. You'll also get a good snapshot of how well the product will perform for your use case.
Best of all, live demos will show you how the AI analyzes data it hasn't seen before in real-time. If it can't work on the spot, that might be a sign it relies more on manual training than it does on "real" AI.
This article was written by Amine Anoun, co-founder and Chief Technology Officer at Evisort, and was first published by Forbes.
Find out how
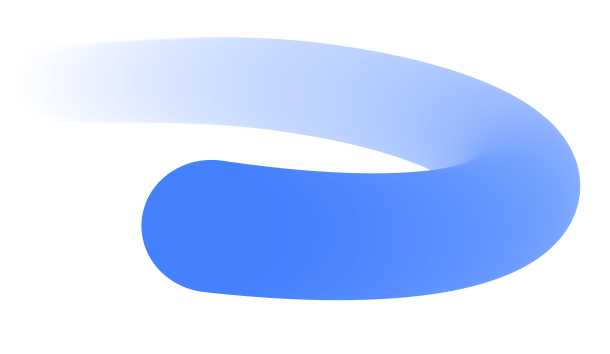
Evisort
can help your team
Test Evisort on your own contracts to see how you can save time, reduce risk, and accelerate deals.