Webinar
Exposing AI Secrets: How to Balance the Use of AI with Data Privacy Concerns
Today’s in-house legal teams are faced with increasingly complex business decisions and newfound challenges, including balancing the usage of artificial intelligence (AI) with data privacy concerns. How is generative AI being used in contract lifecycle management (CLM)? How do you determine whether a large language model is secure? What red flags should you look for when you are considering integrating AI with your existing technology ecosystem? Watch this on-demand webinar with Evisort founder and CTO Amine Anoun and Associate General Counsel Colby Mangonon for actionable guidance on how to evaluate AI and data privacy to:
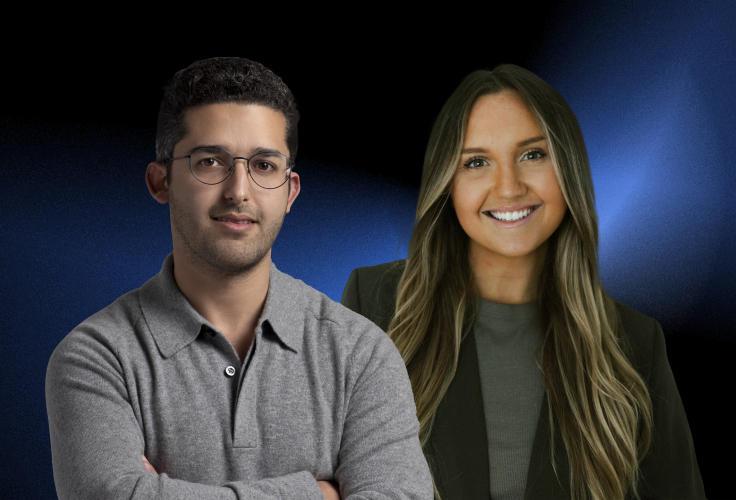
Karen (00:00:06):
Welcome to today's presentation. We are about to begin. Over to you Scott.
Scott Ferguson (00:00:11):
And thank you very much, Karen, and hello everyone. My name is Scott Ferguson, and welcome to today's webcast entitled Exposing AI Secrets: How to Balance the Use of AI with Data Privacy Concerns. This event is brought to you by law.com and sponsored by Evisort. Before we begin, I'd like to go over some basic housekeeping items about the webcast console. This event is completely interactive and features many customizable functions. Every window that you currently see, from the slide window to the Q&A panel, can either be enlarged or collapsed. So if you'd like to change the look and feel of your console, please go ahead. If you have a question for one of our speakers today, please enter it into the Q&A widget on your console. That widget is accessible on the left hand side of your screen where you see the question mark icon.
(00:00:54):
We'll try to answer your questions either during the event or as part of the live Q&A session. At the end, we'll answer as many questions as possible, so we invite you to ask away, and if we do not get to your question, you may receive an email response after today's event. Evisort would also like to remind you that they have additional resources for the audience that you can download and explore. And now for today's topic and the reason why everyone is here today, Exposing AI Secrets: How to Balance the Use of AI with Data Privacy Concerns. Today's in-house legal teams are faced with an increasingly complex business decisions and newfound challenges, including balancing the usage of artificial intelligence with data privacy concerns. How is generative AI being used in the contract lifecycle management? How do you determine whether a large language model is secure? What red flag should you look for when you're considered integrating AI with your existing technology ecosystems?
(00:01:49):
Joining us today to discuss these questions and a lot more is an Amine Anoun. He is the founder and Chief Technology Officer at Evisort, and he's going to be joined by Colby Mangonon. She's the Associate General Counsel at Evisort. And with that Amine, I can turn the conversation over to you to start.
Amine Anoun (00:02:09):
Thank you, Scott. Hi everyone. Very excited to be here. Thank you for tuning in. So we have a very straightforward agenda today. We'll start with talking with the current technology landscape and walk you through different types of AI and AI applications and providers. We'll spend some time talking about potential opportunities and risks with AI, and in particular with generative AI. And then Colby and I will walk you through some practical tips for managing AI and the risks around it, questions to ask vendors, and so on. We'll open it up for live q and a at the end to answer any questions you may have about the topic.
(00:02:46):
So I'd like to first start with an introduction of who Evisort is to put the conversation in context. We are an intelligent contract in software that touches all parts of the contract lifecycle. The first capability that I'd like to highlight is the ability to connect. So Evisort is able to ingest contracts seamlessly by connecting through a two-way sync to a CRM, for example, like a Salesforce or a storage provider that you may have, like a SharePoint, Box, and so on. By ingesting the data, we then analyze it. And that's where I think Evisort shines, is its ability to extract data with proprietary, pre-trained or self-serve deep learning AI to extract data points from your contracts. Those are things like your counterparties, your key dates in a contract, your payment terms renewals, as well as a long list of clauses that you have in your contract.
(00:03:44):
All of that is achieved with proprietary AI. And we don't stop at providing the data. We also make it actionable through the ability to search through your corpus of documents, generate reports on those documents, set up alerts, as well as visualize insights in fully customizable dashboards. We also manage your contracts. So I mentioned we touch the different parts of the contract lifecycle, all the way from drafting, negotiation, approval, and signature with no code workflows. So before we go into the topic today, I'd like to ask the audience here how familiar you are with using AI in your business. So in the poll here, you have four options. The first one is, you're very familiar, you use AI regularly in your work, second one is you're somewhat familiar, you use it maybe occasionally, or you're a little bit familiar, maybe you're new to it or you're starting to explore using it in your work, or fourth option, you're not very familiar and that's why you're here to learn. We'll give a couple seconds for folks to answer.
Colby Mangonon (00:04:54):
I am curious if this has changed recently with ChatGPT and AI being more of a conversational topic.
Amine Anoun (00:05:04):
Yeah, I'd be very curious to see how this answer is different today from comparatively six months ago or six months from now. I think the space is moving really, really fast.
Colby Mangonon (00:05:16):
Absolutely. Okay, we still have some results coming in. A couple more seconds.
Amine Anoun (00:05:23):
Yeah.
Colby Mangonon (00:05:28):
Okay. So it looks like the majority of participants are a little bit familiar with AI and they are exploring the use of AI. So I think this is a timely conversation for us to discuss some foundational framework and evaluation criteria for you looking at those AI tools. With these ChatGPT and GPT4 models triggering this global conversation around generative AI, legal teams and also attorneys that are in firms have to decide how to best balance their use of AI with data privacy concerns. I think it's really important to understand the different foundational AI models before we jump into those data privacy concerns because there is a lot to unpack there and a lot to understand, to know how data privacy will touch all of these different types of models. So Amine, would you mind...
(00:06:24):
Oh, I'm sorry. First, so do we think all AI is equally risky? So if we're talking about every type of AI model that's out there, do we think that yes, all AI holds the same level of risk, no, some AI is less risky, or we're not sure and we're interested to learn? And we will go into what these different types of AI models are and how they are integrated in business tools.
Amine Anoun (00:06:55):
And I can already see some responses trickling in, and I think there is definitely a winning pattern.
Colby Mangonon (00:07:01):
There is, which I find very interesting. Okay, so the consensus here is no, some AI is less risky. So let's talk about the different examples of artificial intelligence, and then we can move on to what this risk profile looks like.
Amine Anoun (00:07:18):
Yeah, for sure. So the idea here is that we think there are a lot of AI applications that are already fully embedded in our day-to-day, and I'd like to walk you through some examples that may or may not be visible. The first one is recommender systems, which is a type of application that deals with recommending content to users. You may see this in your streaming services, for example, like in Netflix where you get recommendations for shows and movies to watch. That's understanding patterns of usage, that's understanding data about you and recommending contents based on that. Another example of recommender systems is if you use search engines, most likely like Google or bank or others, you are seeing ads that use those same types of technologies to recommend ads to you based on your patterns of usage. Another type of AI is speech recognition. This is becoming very embedded in our day-to-day life.
(00:08:18):
If you're using a home assistant like Alexa or you're interacting with Siri on your phone, for example, then it uses speech recognition to parse the information that you communicate to that home assistant, for example. Computer vision is another very interesting application of AI. We see it through facial recognition software, for example, if you have a ring camera. We see it through self-driving cars. That's how they understand the world around us and make decisions on the spot. We're also seeing it more and more in healthcare, for example, as far as medical imaging to be able to analyze x-rays, MRIs, detect certain forms of cancer, et cetera. Natural language processing is another very common, very popular use of AI.
(00:09:05):
If you use a Google inbox, for example, there's an automated spam filter that runs on your emails, scans through all of the text there, and automatically detects whether it goes into the spam folder or into your primary inbox. Another example of NLP is machine translation to be able to automatically translate texts from a language to another. The one that's very timely in that we'll spend a little bit more time talking about today is generative AI and use of large language models, which as you may know, is the field of AI that deals with generating original content, whether that might be text or image, or even audio. We've seen many applications of it and we continue to learn every day, but examples are writing marketing blogs. We've seen it being used in support chat bots, even email drafting for sales outreach, for example.
(00:10:02):
So the point here is that we are already embracing AI in a lot of aspects of life, and I think different applications have maybe different levels of maturity as far as the security and the data privacy considerations around them, and that's what Colby and I will dive into in the rest of the call today.
Colby Mangonon (00:10:20):
So as you can see, all these examples likely include some form of data ingestion and retention the levels of risk exposure both individually and as an organization very significantly, but a very important thing to understand is that the models themselves are not any more risky than another. So generative AI is not inherently more risky as a model. It's more the data retention, the data ingestion, and the data training policies that exist around these models that may change that risk profile.
Amine Anoun (00:10:54):
So I want to spend a little bit of time talking... We talked about the types of ai, but I want to put it in the context of contract management and talking about some of the applications here, because as we go later through the different data privacy concerns with those, we'll come back to these applications and kind of assess the levels of risk. The first one is computer vision is very widely using in contract and document management in general. And the example I'd like to give here is optical character recognition or OCR technology, which lets you parse the image of a document in maybe a PDF format or PNG, JPEG, or so on into machine readable text so that it can be fed into maybe a downstream natural language processing that's able to read and understand the text. So natural language processing is obviously the bulk of the application of AI in contract management.
(00:11:50):
That's the technology that reads text and performs a certain number of tasks, such as data extraction, data summarization. Classification of documents is obviously one that we use very widely at Evisort, but is also used across the contract management space. Generative AI is the new technology that's being used in contract management. And when I say new, it's not necessarily a new advancement that happened in the last year. It has been here for a couple years already, but we have seen advancements in the level of performance and accuracy that now makes it a valid use for enterprise use cases. And generative AI can have a lot of different applications in contract management. A few that I'll mention is the natural use case of drafting a contract. That's kind of the traditional use of generative AI's generating original text. And so you can rely on generative AI to some extent to take a first pass at drafting maybe a clause.
(00:12:49):
You can give it an instruction to, hey, can you draft a termination for convenience clause that let me terminate with a 30 day notice? And it gives you starting language for you to review. It doesn't claim to be able to do that fully automatically. It's not going to not require any level of review. I think we're very, very far from that, but it can definitely be an assistant and a way to augment drafting of clauses as you're going through your daily workflow. The other use case that's really interesting with generative AI and contract management is ability to modify the text in a contract that's being negotiated. So an example here is let's say you start from that termination for convenience clause that allows for a 30 day notice, but maybe your playbook says that you have to require a 60 day notice for a counterparty to be able to terminate.
(00:13:42):
If we have knowledge or access to that guidance or that company playbook that you may have, then you can imagine that generative AI can automatically go into the clause that's being recognized with NLP capabilities and generate text, generate red lines automatically to make it fit with your company's playbook. And that can scale to a lot of different clauses and positions as well. Another use case I'll mention for generative AI is the data extraction itself. We have seen a lot of promise in the ability of generative AI, and not just open AI models like ChatGPT or GPT4, but many others by other companies. We have seen promise in the ability to extract specific fields or clauses from documents through a question answering mode or basically by parsing the meaning in the paragraph for the document and being able to surface that information to the user.
Colby Mangonon (00:14:37):
Okay. And then as we move to what other use cases there are for generative AI, I would love to know a little bit more about the background. I think it's great context to understand how the model is trained, what pre-training means, what fine tuning means, and really how that works into this data privacy context.
Amine Anoun (00:14:56):
Absolutely. So generative AI relies on the concept of large language models. These are deep learning models, particularly using transformers that are trained on a very, very wide corpus of data. What that means is that it's trained on publicly available sources of data, for example, news articles, Wikipedia, social media interactions. The list goes on and on. And that generates at the very large scale a large language model that a user is able to interact with to be effectively mimic that behavior that's seen in the language drafting in those news articles, social media interactions and so on. So that's the concept of pre-training a large language model. However, it doesn't stop there. There's also the concept of fine tuning. And so what fine tuning consists of is starting from what's called a backbone model, so that's that base model that has been trained on a very large corpus of data and usually is more geared towards generalist or horizontal applications, to fine tune it to a specific vertical use case.
(00:16:07):
So in the case of legal, for example, you may think of it as starting from a large language model that's really geared to understand English or some language generate text for general use cases, and then training that on contract data to be able to perform better at specific tasks that are relevant to legal professionals. And I'll go a little bit into what are those types of tasks that generative AI can accomplish. Question answering is the obvious one that we've seen gain a lot of popularity with ChatGPT, which is the ability to interact with generative AI as a chat bot that answers a vast a variety of questions. The second one is text generation and summarization. That's also a very classic use case of generative AI. I mentioned earlier the examples of drafting a clause from scratch, as well as drafting a marketing copy or an email. That's something that generative AI is able to accomplish.
(00:17:05):
It can also summarize text, so you can feed it a certain length of a document and ask it to summarize the information and hit on the specific key points in that, and it can do so pretty successfully. The next one is data organization and classification. This is a very interesting use case of generative AI, where if it has access to a corpus of documents, it can, through field and metadata extraction, understand and assign tag to a different documents so it can organize them in a certain way. So theoretically, if it has access to a corpus of documents and it's able to classify those documents into the types of contracts or the types of law that they represent, it could group them into folders based on that information and help users organize their data moving forward in an automated and real time way.
(00:17:58):
Data extraction analytics is also another very strong use case or task that generative AI can be tasked with. And in particular, now that you have that folder structure with your different documents and the different tags that were extracted by generative AI, you can start visualizing that in dashboards that update in real time. That is very powerful, to have that sort of view on your documents at any point in time. Also mentioned image generation and manipulation. We've seen a lot of image synthesis models like DALL-E. There are a couple others that are able to generate original images and content. And there are a lot of interesting legal questions there, I think, around intellectual property and others that I'm sure Colby would know a lot more about, but it's a very interesting use case to be able to generate an image, and even thinking about documents, to be able to generate tables logos and goes beyond that.
(00:19:02):
So at this point, we've spent some time talking about the AI landscape, understanding the different applications and use cases in contract management, and also more broadly I'd like to shift the attention to thinking about the data privacy concerns. And the question here is which of these could be a data privacy red flag? The first one is no data retention policy. The second one is the inability to opt out from using your data to train models. The third one is models are being fine tuned with personal or sensitive data, or all of the above. So I invite the audience to submit your responses, and we'll share the answers with you as they come in.
Colby Mangonon (00:19:57):
Okay. It looks like the overwhelming amount of answers are all of the above. So let's discuss a little bit about these data privacy red flags, the first being no data retention policy. And it's important to note that the API integration of the open a AI models, such as ChatGPT and GPT3, when integrated using an API, they do in fact have a data retention policy, but there are other models that are available out there that may not have a data retention policy. And so that's something that would be a red flag when you are evaluating a vendor and the third party AI provider that they're using. The second is the inability to opt out from using your data to train models. So this was a concern. And we'll discuss this a little later when we talk about GDPR.
(00:20:49):
This is a concern about your data deletion rights, data correction rights, and also, there are concerns about data leakage. So with the ChatGPT, this larger public model, there is a possibility for data leakage. We have seen vulnerability attacks on some of these LLMs that allow them to extract data from the greater data that's underlying the model, what it's been trained on. And so if you can't opt out from using your data to train those models, that is a concern that you may want to consider. The third is models are fine tuned with personal and sensitive data. And this is actually an interesting one. It's tricky because there is a possibility for the models to technically be fine tuned with personal and sensitive data, but they're not. So if your data is brought into a vendor's platform but that vendor then de-identifies the data, removes and strips all the personal and sensitive data, anonymizes and then aggregates that data, then you don't have as large of a concern as if the model is actually be being trained with proprietary, personal, or sensitive data.
(00:21:58):
So we will move up onto this visual representation of data privacy framework for generative AI. These are all concerns that you may want to evaluate when you are speaking with a vendor. I'll just go through some of them. But data input, the data that's being output. Your data output, there are additional ethical concerns. It's not necessarily data privacy, but there may be some CCPA or GDPR concerns with that data output, your data retention policy, the security framework around your vendor, and then as well as the third party provider that is providing them with that API integration, the data training of that underlying model. And then you'll see some others on here like compliance, IP, and the legal concerns as well.
(00:22:49):
When we talk about the GDPR, CCPA, and CPRA concerns with generative ai, the first large concern we're looking at is the data deletion concept. And so there is a codified right to request this deletion or correction of personal information with the ChatGPT, which it has now been remedied, there is not data training on this public model. That's what they're saying, is that OpenAI is no longer training the public model with the data that has been inputted by users, but there is a question and the concern of if the model is fine tuned on that data without consent, is there a possibility to request deletion or correction of personal information? This also goes to the pre-training of the model. So many of these models have been pre-trained on webs scraped data. When we look at that webs scraped data, is there a possibility to request deletion or correction of the information that has been scraped from the web?
(00:23:49):
So that's the main data deletion concern. When we're looking at these regulatory GDPR, CCPA, and CPRA concerns, we also have to look at the consent. So GDPR requires that there is consent or legal justification to collect or provide personal or sensitive data. Most of the time when you are interacting with a vendor, you are providing that consent. And so when we're talking about your evaluation of a vendor use of generative AI, this is not as much of a concern as when we are looking at the underlying pre-training of that model and that web scrape data, whether or not they had the consent or legal justification. This is something that was raised by the Italian regulator, which we'll discuss in a minute.
(00:24:34):
The third is the use case. And so when you're looking at vendors, you probably won't have as big of an issue here because the integrated a API access of these models like GPT4 or CHatGPT, typically your vendor is going to be complying with GDPR or CCPA in their provision of services, but it's never a bad idea to make sure, check for yourself that they are complying with CCPA and GDPR in the way that they're integrating these models and making sure that there's no violations there that would be an issue for you. The last thing I want to discuss is Italy and also just this broader EU landscape for ChatGPT. So as many of you probably know, Italy did do an all out ban of the public version of ChatGPT. Now, there have been subsequent discussions about ChatGPT and about this regulation. In those discussions, they have made it clear that they don't intend to punish people who use an API integration of OpenAI. They are just wanting to make sure that open AI's models are in compliance with GDPR principles.
(00:25:46):
OpenAI has released statements stating that they are going to allow for data deletion concept and that they're going to try and remedy this to remove the ban in Italy. But one of the main concerns that was raised was the pre-training process of LLMs, so that underlying web scraped data that was brought in to train the LLMs. Why this is interesting is because many, if not all LLMs, and many, if not all other types of AI models likely include some form of web scraped data for their pre-training process. And so if we have an EU regulator saying, "We are going to absolutely invalidate the method that we've trained all of this AI that exists," we would essentially be removing most of the AI that's out there on the market today.
(00:26:38):
And so I do think that we're not going to see that level of regulation. I think what we're going to see is a move towards the data retention policies, the fine tuning process of the data that is submitted by users in both the public and the private models, and also more of a responsibility for the output of what these models are creating from ethical concerns. And then just in general of what ChatGPT is actually generating, we are going to have a little bit more regulation there. So there's two levels of concerns. There's the underlying web scraped data that is ingested by the pre-training process of the model, and then there's the secondary layer, which is the fine tuning process and how the integration of these APIs are being used by vendors, what data is training that model, et cetera.
(00:27:34):
So we provided a couple questions to ask when evaluating generative AI. I want to discuss these six in particular. The first is what information are you providing to the LLM? So if you're looking at an LLM for the use of publicly facing advertising information, your evaluation would likely be very different than if you are integrating with an HR platform, and there's a possibility of personal and sensitive information being ingested. So that's the first thing that I think is, number one, the step that you need to consider on how much your data needs to be protected and what these other profiles need to look like for you to feel comfortable. The second is what is your use case?
Amine Anoun (00:28:19):
If I may, on the first question, I think there is an interesting example here on... Tying back to the applications of generative AI and contract management, I gave the example of drafting a new clause with an LLM with a kind of generic instruction of draft determination of convenience clause. There is nothing proprietary about that type of instruction. So that's a good example of what doesn't present a concern here from a data privacy and security point of view. On the other side, if you're sending an entire contract to a third party, that third party does not comply with the right data retention policy or data deletion policy, or you talked also about web scraping, the ability to take that data and use it to train other models. There are so many considerations there. Then you are in a risky area in that case, and that's something you want to take think about twice when approaching that vendor.
(00:29:15):
So it is very important to understand exactly how that the data is being used. And it may be okay if you're not feeding proprietary data and you get a lot of value out of it, but it may be very detrimental if you're sending that type of sensitive data to get the output back.
Colby Mangonon (00:29:32):
Absolutely. And the second question is, what is your use case for the product or service? And why this is important is that the models themselves also suggest this, but there are ethical requirements and additional regulatory requirements for certain industries. And so we are seeing that specifically in legal, medical, and financial industries, that there needs to be a level of supervision for the output of this generative AI product. It's probably a good idea to have a human review of this output either way, but in those industries, it's specifically required. And that's required by the model itself, and then also by ethical obligations that we have. Number three is, is the LLM integrated by an API or is it public? And so the API...
Amine Anoun (00:30:20):
[inaudible 00:30:21] here quickly because I see a question coming in of, what does an LLM mean? In this context, LLM is a large language model, which is the basis of how generative AI works.
Colby Mangonon (00:30:32):
Yes, correct. So if the generative AI model, if it's integrated, are they using an API integration, or is it a public use? The reason this is important is that open AI in particular, and for you to understand if they're using a different model other than ChatGPT, or GPT3 or GPT4, what does that API allow? What are the additional data protection policies that they have? OpenAI is allowing for a separate instance and an opt-out for data training, also a data retention policy that's 30 days, and that's what the API use. However, the public use of ChatGPT is not... It doesn't have those same protections. And so I think it's really important to look at those two factors separately. You may want to have a corporate or business policy that states that employees are not allowed to use the public version of ChatGPT or giving factors or parameters for their use. And then the second is an integrated vendor use, which is likely going to be through an API.
(00:31:36):
Number four is the data retention policy, which we touched on a little. And that's important because obviously we all have data retention policies that we have to comply with. We have obligations to our customers, and so we want to make sure that the data retention policy, first of your vendor, but also of the underlying third party API provider, that they rise to the level of your requirements. And then number five is what are the InfoSec and data processing policies. And so, again, you'll need to look at your standard procurement process of looking at your vendors' InfoSec and data processing policies, but you also need to really dive into what the third party provider has for InfoSec and data processing policies to make sure that you trust and feel comfortable with how they are processing your data and the security framework for their processing and ingestion of this data through that large language model or the generative AI model.
(00:32:35):
And then number six is, will your personal or sensitive data be used to train a publicly available model? Again, when we see this, a API use of ChatGPT or GPT3 or GPT4, if it's integrated through the API, we do have the ability to opt out, and we also did discuss earlier that de-identification, and then aggregate process, the anonymization and aggregate of the data that can be used by providers, and that may actually give you more protection, but you just want to make sure that you're not having the model ingest personal or sensitive data, and then it's being released to that public model.
(00:33:15):
Okay. We have a lot of questions coming in, which is amazing. We have one more slide, and then we're moving on to the Q&A. So I'm just going to move through that, and then we can discuss all of these great questions. So we will have a PDF available, but for now, we do have this up on our blog. It's a checklist to assess data privacy policies for all AI providers. It discusses particular questions for each of these factors in the framework, and it also has factors that are specific to generative AI. So this is available to you. I think we may be posting the link as well, but it will be available on our blog. And so this is just a great resource for you to use when you were evaluating a vendor, some great questions that you can ask to make sure that you and your business are protected. Okay.
Amine Anoun (00:34:10):
Awesome. Yeah. We definitely got a lot of questions during the presentation, so we'll have time to answer them. And Colby, I think this first one is for you. The question is, how will the EU regulator's decision impact use of generative AI?
Colby Mangonon (00:34:26):
Okay, great. So like I said, there have been other countries weighing in on Italy's ban. There have been additional countries weigh in such as Germany, France, Spain, and they did address the fact that they don't intend to punish the integrated API use of this large language model, but they want to just make sure that there is compliance with GDPR. We are seeing kind of a move away from... From France and Spain, we're seeing a move away from the underlying pre-training and the webs scrape data into more about data leakage and bad actors and what this fine tuning process would look like. We're also looking at what the data retention policies are, data training policies, and then the other focuses that are important. But maybe not as relevant are the age restriction mechanisms, and then of course IP protection. That's a really big hot topic here as well.
Amine Anoun (00:35:27):
A related question that we got is, how does Italy's decision correspond to the UK EU expansion or rights to scrape the web?
Colby Mangonon (00:35:36):
Right. So it's a very hard question to answer because we all know that we can't necessarily predict what's going to happen, right? But I think that the web scraping is a really interesting... It's a contextual conversation, right? So there's this really interesting data privacy principle that it's about context. So even though I post something publicly on Reddit, it doesn't mean that I contextually wanted it to be allowed to train a public model. Was there a legal justification or consent? I think it could be argued, yes. So I think that there's a possibility that we will see large language models get around this and not really have an issue because there was some level of consent, and that the contextual obligations maybe met. There also is something to be considered when you think about, okay, if something was posted online as a template and it was used to train the model, does that still remain in the same contextual sphere? So would you be able to have that be a legal justification or a contextual justification?
(00:36:46):
I think when we look again at this web scraping of the underlying data, we're not going to see them invalidate every type of LLM or every type of AI. So I think they're going to find more particular rules and regulations on how we can use those models, but it's also interesting to note that these generative AI models may be able to remove this web scraped data and retrain the models without it. So if that's a possibility, even if we do get to that extent, we may see really no issue with these models continuing because they may be able to remove that data itself.
Amine Anoun (00:37:25):
Yep. I'll take the next question, which is an interesting one. We're seeing many vendors build a simple wrapper around open AI's chat capabilities. Do you see that as a successful approach and how can we differentiate these solutions? I think this is a very relevant question. We're definitely seeing a lot of that. And I think the value really is, if you're just putting a wrapper beyond OpenAI, you might as well connect to it directly and you have more control into how you're connecting with those types of models through the API integration. Like Colby mentioned, and you have more control into the data you're sending, how it's being processed, and so on. My bias here is towards creating value on top of these types of connections or wrappers, and particularly around vertical use cases. So if it's simply making the chat capability of OpenAI available and embedded within another application, that doesn't necessarily carry a lot of value.
(00:38:25):
But if you think back about the example I gave around automated redlining and a contract, the ability to understand your previous positions on the documents that you have agreed to your company's playbook and connecting that in the same system to the generative AI capabilities that are available out there or that are proprietary to that vendor is a lot more powerful. And I think we're likely going to see that in the industry. We will start moving more and more towards vertical applications of generative AI. And so I think ultimately, the wrappers around open AI are going to almost disappear maybe and make room for more vertical value driven applications because we've seen that actually with a lot of AI applications in the last five to 10 years. Like IBM Watson started with a great promise, for example, of delivering value on the ability to work well actually in legal, in health, in a lot of different industries. And what we saw is that there were a lot of vertical applications in each one of these industries that was able to go beyond that value.
(00:39:36):
And I think OpenAI is a great start for the generative AI conversation and quality of the models that are available out there, but I can see a lot of opportunities for vendors to take that and add that last mile of value to users.
Colby Mangonon (00:39:52):
Okay. And this is kind of related, but how do you see the generative AI space evolving?
Amine Anoun (00:40:00):
Yeah, another very difficult one because it really changes every day. So couple thoughts here. First one is I think there's a lot of push, by both academic institutions and companies, to invest into generative ai. So it is going to continue to move very, very fast, and I think there is a very big push towards open sourcing these types of models. Going back to the point about building vertical applications, that these vertical applications are going to take advantage of the open source versions of AI that's available out there. We're also starting to see a lot of solutions being more and more open for commercialization, so I think there is a general kind of democratization of generative AI that's happening in this space.
(00:40:49):
I think, again, a very clear direction that generative AI is taking is building very specialized niche vertical solutions that perform better against the kind of general benchmarks. And the general benchmarks, don't get me wrong, are very, very important, they are the ones that are unlocking the value that these vertical applications are able to deliver on, but I think the race to have the best generative AI model for general use is going to start reaching diminishing returns, and then we'll start seeing a lot of value in health, in legal and a lot of other spaces, marketing, and so on.
Colby Mangonon (00:41:31):
Okay. And this question is essentially, how will the confluence of AI models deliver an output that will keep my organization from litigation? More specifically, how is AI going to be a multiplier of efficiency of administration of the organization without risk of litigation by using it? I think this is a really interesting question, but I do think that what we've seen in the CLM space is that there is a move towards building models that are built off of your existing playbooks, and off of your existing rules and parameters. And so what generative AI can do is essentially take your playbook and then ingest a third party contract, redline that to match what your playbook terms say, and then there would have to be a human supervision aspect of that. And so when we are using your underlying playbook or your cause library to inform the decisions of the AI... And this goes back to what Amine is saying. If we're looking at a straight use of ChatGPT, will that be great for contract negotiation?
(00:42:35):
Probably not. But when you're looking at it, when it is integrated with a proprietary AI that is trained to recognize and read clauses and understand what a limitation of liability clause looks like, it knows better where to ingest those terms that exist in your playbook. And so that's where we see that additional layer of protection. We do also think that there will always be attorney supervision because it's ethically required of us. If you had a paralegal that was drafting documents for you, you would need to review those. If you had a law clerk, no matter how brilliant they were and how much you trusted their judgment, you still would need to supervise that. So it's more of an efficiency tool than it is replacing lawyers and making our jobs obsolete.
Amine Anoun (00:43:26):
There's another interesting question here. How do you draw a line between pre-training data that might include PII? How would someone know? And how can you take down something that's already been trained? I'll take a stab at this, and Colby, curious if you have thoughts on this as well. But there are technologies today that pretty reliably detect the presence of PII information in a document. And so I think you need to overlay generative AI on top of that to make sure that it's not ingesting anything that's sensitive or can qualify as PII. This is not new, actually. A lot of the AI that has been around for the last few years in the computer vision NLP applications that I talked about has to be built on top of that, because even then, you want to make sure that it's not touching the PII information in a contract, and I think the same applies here with generative AI. So the second part of how would someone take down something that's already been trained, it's a very complicated question from a technology point of view.
(00:44:30):
If the data has been aggregated, anonymized, de-identified correctly, as Colby described, then ideally there wouldn't be a need to do something like that. But if there is a need, if there is data leakage or anything, then maybe that risk is higher with how generative AI is being used, then removing that data from the training means effectively training a new version of the model, which can be very expensive. So I think that's going to be an ongoing conversation between basically the technology space and the law and regulations to find a way that kind of minimizes the impact on a vendor to retrain a model that can be very expensive, very costly, very time consuming to respond to a specific request from a user, and it may end up being an opt-out early on in how the model is trained, and not necessarily after the fact the ability to go back and delete that data. But Colby, I'm interested to see if you have any views on this point.
Colby Mangonon (00:45:35):
No, I agree with you. I think we are seeing an expansion of data deletion mechanisms. Like I said, OpenAI had a statement that said that they were working on data deletion for ChatGPT and that public model. I think that it's definitely something that is required under certain regulations, but I think that removing that from the underlying trained data there isn't... You can do a data deletion request or a DSAR, but you know really wouldn't know whether or not a model has been trained on your data unless you did a data deletion request for every large language model provider. I think some of the more concerning applications of that PII being ingested would be on the fine tuning side, rather than the pre training side, and also in which application it's being used, and when we see the extent of what data leakage and vulnerability attacks can do. So it's a complicated question, but I think Amine did a really great job answering it.
Amine Anoun (00:46:45):
There is another question here, and I'm definitely not surprised to see it, which is, do you see generative AI as a threat to the legal profession? Colby, as the legal professional here, I'll let you answer this one.
Colby Mangonon (00:46:55):
I may be biased here, but I actually think it's wonderful. I don't see it as a threat to the legal profession at all. I see it as a tool that can be utilized. I think it can increase efficiency. I've seen what generative AI can do in terms of a third party contract coming in. I just think about it's Friday afternoon, you have a third party contract that comes in, it's 76 pages, and you're going to be working all weekend to get it done. With generative AI capabilities, you can run the generative AI on the contract based off of your clause library or your playbook, and it will automatically redline out the clauses to be in compliance with that underlying playbook. And now instead of going through and doing all of that manual review and redlining, I can review the redlines that exist and modify them, remove them, decide what I want to do, and that gives me more of the strategy back.
(00:47:54):
It gives me more of the deep analysis back, as opposed to the manual labor of going through and deleting words, changing an on-prem services contract to a SaaS contract because the third party contract doesn't really relate to our services. I think with any tool, you're going to have pushback, and legal is usually a slow industry to evolve with technology. But I really think that if we embrace some of these tools within this framework of understanding data privacy concerns and how we can protect our businesses, I think it's something that can make us even better attorneys. So I don't actually see it as a threat, but Amine, I'd be curious to know, from a development standpoint, if you have any thoughts.
Amine Anoun (00:48:41):
Yeah. Actually I had point about what you said. You mentioned during the presentation earlier the importance of defining the use case for using generative AI. A lot of generative AI is not permitted to be used for certain applications like legal, unless there is a human review element. That's also another example of why the legal professional is still needed as part of that process, and I also don't see that going away anytime soon. There is technically liability with someone trusting technology with making an important decision if they're not reviewing that information, and I don't think we're anywhere close to that. There is a lot of talk about how accurate degenerative AI models are today, but there's also a lot of talk about how they can hallucinate and make up things that obviously we would not want to have bypass a human legal review.
(00:49:38):
So from that perspective, yes, I think anyone that's implementing generative AI in a software that's been used by legal professionals should be thinking about the importance of having the human element and the human in the loop in making the decision on top of that. And that that's also how we are thinking about it at every sort. So the examples I gave around class creation, data extraction, the ability to redline automatically your language to fit a company's playbook, all of these should be able to be overwritten by a user. So it's a way for them to augment their work. It quickly gets you the answer. And maybe it's a repetitive task, like you said, that you just want to get done quickly, but you have to have the ability to review it and edit it as the last step.
Colby Mangonon (00:50:29):
Okay. And I see here, can you share how corporations are managing the use of ChatGPT and/or other similar open ais by their employees? And I assume we're talking about the publicly available models here. The most common two forms that I've seen of this are, one, an all out ban. So they can use it for personal reasons, but on an employee laptop or in the context of their work, they are not allowed to use it. I'm not saying I necessarily agree with that, but that's what I'm seeing as a trend. The second is that they are permitted to use it with a certain set of parameters, like taking out personal and sensitive information, and that when they do draft something using ChatGPT, that there is a disclaimer that says which portions of this message, email, blog post, et cetera, have been drafted using ChatGPT.
(00:51:19):
I definitely think it depends on your industry, it depends on what the output is. What are they using it for? If they're using it for a LinkedIn sales outreach, then maybe you are less concerned, maybe not. But if they are using it for something that is publicly tied to your company, like a blog post, or using it for communications in financial industry, medical, legal, then there may be additional concerns that you should think about. But I do think it's important and probably very smart to have a policy for your employee use of ChatGPT while on the clock.
Amine Anoun (00:51:56):
Yeah, I would 100% agree with that. And even from my perspective, we have obviously an engineering team at Evisort and we had to address this. And I think having access to ChatGPT or similar technologies for our company can be a real productivity booster, so we definitely want to take advantage of that. But what we can't have is submitting proprietary information into these engines. And you may have seen the news about Samsung and engineers submitting a proprietary code into OpenAI. That's definitely what you don't want to happen. But if an engineer is asking ChatGPT, "Hey, can you create a script that does X, Y and Z?" that engineer is not submitting anything proprietary, they're not submitting their code, but they're getting a productivity booster in their day. That may be a good thing for a company to consider for their employees. If they're submitting code and asking it, "Can you modify it to debug something or make it easier, faster, more optimal?" that's a concern because now that's in the hands of OpenAI, and we go back to the conversation we had earlier about deletion, data retention, and so on.
Colby Mangonon (00:53:06):
Okay. And this is an interesting one, but do you have an opinion or view regarding whether a company's failure to review generative AI output before presenting or using that output to deliver services to the company's customers could be considered reckless tort behavior, so as to render a limitation of liability clause, binding the customer unenforceable. Does the area of business the company operates in affect your opinion or view? I do think in a sense the area of business does affect that opinion, because like we said, it's actually a violation of the model's use license to use legal output, medical output output that determines human life in some way or financial industry output without a human overview. So that already is a liability that you're looking at. But also from an ethical concern, if you're providing legal services, of course you're going to want to have human review of that.
(00:54:00):
And I think it really depends on what the output of those services are. So if we're talking about our generative AI model in a CLM space, which is going to use the integration of a clause library to generate AI and provide that service... So the generative AI service that we allow for it will redline that document. I wouldn't say that that we're not having our own engineers or customer success employees review that output before it's given to the customer. I don't think that that is something that would rise to the level of liability. But if you are saying that you are an advertising agency and you're creating unique content, and you're using ChatGPT to create that content and you are not giving them unique content because it is something that is an iteration of what's already been out there from a ChatGPT use, which we have seen this happen, then I think that that would rise to a liability.
(00:54:57):
So it really depends on what the integration looks like, what the service you're providing is, and if it's something I would assume that is already done by an AI model in some way. So our proprietary AI is already doing data analytics and extraction in our contracts. That's something that our customers are comfortable with, they're aware of. They actually prefer that word data agnostic. And that way, we're not going in and looking at what they're doing to their contracts and making sure, monitoring them. So it's definitely a deep dive. You have to kind of look at all the different factors, and then what your service is and what business you're providing. Let's see. Do we have any others?
Amine Anoun (00:55:49):
Yeah, I see a question here around the ability to assess the quality of an LLM and the accuracy. T question has come up a lot as a lot of LLMs are being developed every day, and there isn't, I think, a universal framework for evaluating the accuracy of an LLM yet. A lot of it is being done with kind of ad hoc queries and qualitative assessments. There are benchmarks of data sets that have existed in LLP in particular for the last few years, where academic papers look at the accuracy levels against a certain type of task. There are purposes of questions and answers and ways to kind of automate that assessment, but it doesn't represent the full capabilities of generative AI yet. It certainly is not going to be a good reflection on how generative AI is going to perform on the examples we talked about, as far as extracting data from contracts, classifying them, creating clauses, and how do you even assess the quality of the clause that's being created by generative AI?
(00:56:57):
So it's an interesting question. It's actually one that we're solving right now [inaudible 00:57:01] sort as well of how do we automate or semi-automate an evaluation framework as we continue to dive deeper into generative AI. But I do think that that's also an example of why legal professionals are really helpful here with understanding different considerations with generative AI, is we need to rely on legal professionals to understand what represents the quality of an output to a legal question or legal drafting, and so on. And I think we're going to start seeing some metrics being defined and becoming a bit more universal and shared. And all LLMs will be basically benchmarked against those, but it will be sometime before we get there.
Colby Mangonon (00:57:42):
Okay. And then last question, and I'm actually curious about your opinion on this too, Amine, what's your opinion about marking or delineating AI generated output versus non-AI output?
Amine Anoun (00:57:56):
That's a great question. Yes. I think it really depends on how you're using that output. There isn't maybe a black and white answer to this, but I can think of a lot of use cases where it is important to understand if that's something that has been a manual input versus AI generated. In general, what I feel very strongly about is that anything that's AI generated can be [inaudible 00:58:24] by a user. That's very important. We should not let AI be fully in control of an output or a decision. And so there has to be that path going from an AI generator to a non-AI output for any system that exists. It's something we've thought about at Evisort also, a lot of creating that differentiation. And what we've actually learned is that in the review use case, so if the use case is performing quality control on the outputs of the AI, that it actually biases professionals today to look more at the AI because that's a new technology that we don't necessarily trust and maybe forego the review of the manual manually input data.
(00:59:10):
And that can actually defeat the purpose because many times, the AI accuracy will exceed that of manual review, and we have seen that on data extraction in the last few years. So it's a bit of a double-edged sword of how it's going to be used to have that demarcation. But in the context of generative AI, it is interesting to know whether that something has been generated automatically. And I'm sure you're seeing now a lot of blogs and news articles that start with, "This has been written by a human," which is a really interesting kind of consideration that no one has thought about before.
Colby Mangonon (00:59:46):
And we are out of time. I will hand it back to Scott to do a little bit housekeeping at the end. Thank you so much.
Scott Ferguson (00:59:54):
Okay. Well, thank you, Colby. Appreciate it. And that is all the time we have for today. As we mentioned, we want to thank everybody for joining us, and we hope this event was useful to you. Once again, thank you to our speakers, Amine and Colby for their insights, and Evisort for their sponsorship at today's event. As a reminder that Evisort has some additional materials that you can download and read when you get a chance. And if you missed any part of this presentation, remember, it'll be archived shortly law.com so you can refer to it again or send somebody else to our page there to view to. And while you're there. We always encourage you to look at other upcoming and on-demand presentations. With that, thank you again everybody for joining us, and have a great rest of your afternoon.
Find out how
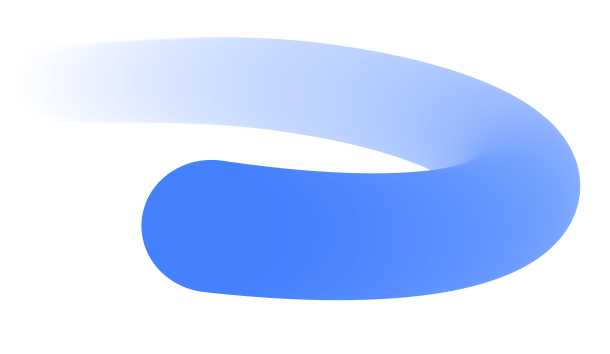
Evisort
can help your team
Volutpat, id dignissim ornare rutrum. Amet urna diam sit praesent posuere netus. Non.